All scientists know that data is central to scientific progress. The challenge for modern scientists is searching through and analyzing the huge volumes of data being produced to uncover new insights. It can be a very time-consuming task because this data is usually sat in disparate instruments, stored in passive warehouses, buried under lines of complex technical code, or just severed from the scientific process. These scientific data management challenges make a scientist’s life much harder than it should be by putting up persistent barriers between the scientists and a contextualized understanding of the data.
In this blog we explore some of the reasons today’s approaches to research data management are falling short, and how scientists can overcome them with a unified, standardized, and science-aware approach.
Where today’s approaches to scientific data management fall short
There are a multitude of obstacles scientists face in realizing the full potential of their organization’s data. But broadly speaking, these challenges can be categorized into three larger buckets:
Scientific data fragmentation
The average pharma or biotech organization uses dozens of different systems and hundreds of instruments. Each of these instruments generates a vast amount of data, and all scientists know the pain of moving this data into analysis software, LIMS or ELN to capture the results. When you multiply this across many instruments and software tools, it is easy to see how data can get fragmented across an organization.
And the problem gets worse if scientists need to analyze data from multiple instruments for a single experiment. They need to collate data from all the instruments they’ve used, standardize it, and then place it into a system where they can finally analyze it. As a result, it can take hours or more for scientists to extract a single, meaningful insight.
Standardization
The second challenge is one of standardization. While some organizations go one step further by adopting data lakes, warehouses, or lakehouses to bring their data together, the shortcomings of these approaches quickly become clear when scientists attempt to use them. A data lake can bring large volumes of data together, but the data retains its original format, and it isn’t designed to be easily accessible by a scientist.
Similarly, a data warehouse places scientific data in one central location. This data is structured, making it easier for scientists to view and compare, but the cost of maintaining a data warehouse is exceedingly high, and it severely lacks the flexibility and scalability demanded by modern science. Once an organization’s science and its associated data evolve, scientists are right back where they started, with a massive programming feat standing between them and their next big breakthrough.
Data lakehouses have the benefit of combining structured and unstructured data, and they offer greater scalability, but they are equally costly to maintain and still fail to deliver optimal flexibility and full scientific context.
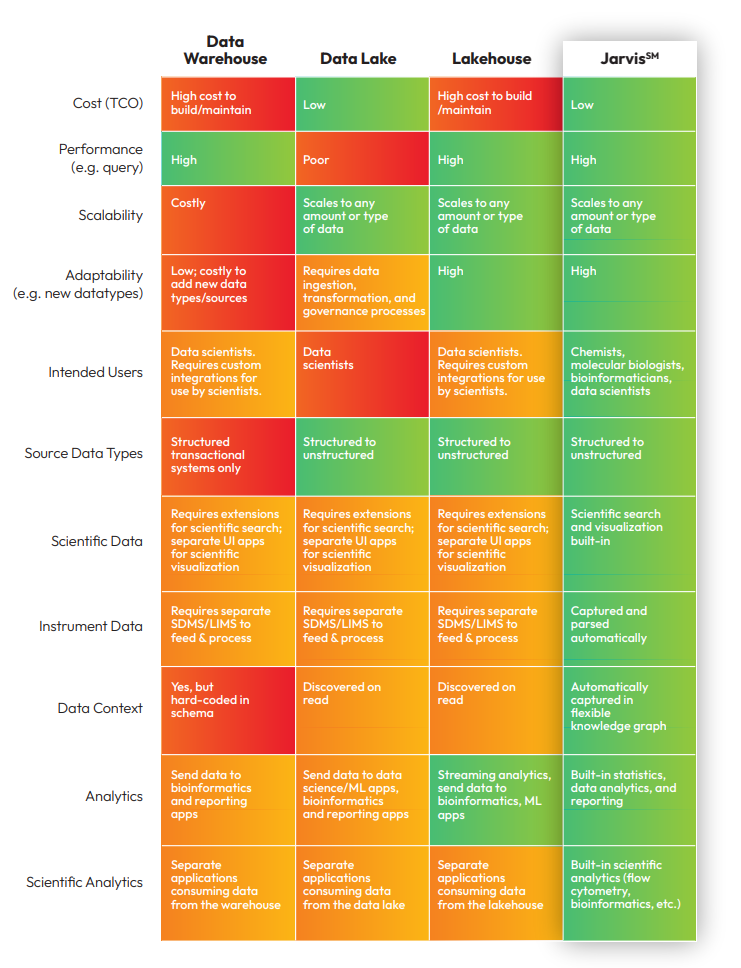
Data consolidation ≠ data utilization
That brings us to our third challenge: utilization. Data lakes, warehouses, and lakehouses are simply places for raw data storage — they can be used by any organization, regardless of industry, and don’t conform to the FAIR guiding principles for scientific data. They do not recognize scientific data as distinct from consumer product data, or industrial manufacturing data, or any other sector’s data for that matter. Because they are not scientific by nature, they do not come equipped with the tools and capabilities scientists need to transform complex scientific data into tangible insights. This makes it difficult if not impossible for scientists to derive the full value from their data.
And while there are scientific data management systems (SDMS) that are designed to take data from lab instruments and software platforms, they don’t really address the issue. The data they collect still ends up in a data lake, warehouse or lakehouse. The fundamental point remains that no matter how you’re collecting and storing your scientific data, unless your approach provides tools for scientific analysis and utilization, it isn’t helping scientists.
When you break down the options scientists have available today, it quickly becomes clear that data consolidation isn’t enough to unleash the full potential of an organization’s scientific data. Rather, it is but the first step towards accelerating discovery.
What is a science-aware approach to consolidating scientific data?
To solve the challenges of fragmentation, standardization, and utilization, scientists require an approach that is truly science aware.
The ability to simply find data isn’t sufficient for scientists—although having their data all available in one place is an important starting point. To extract meaningful insight and drive discovery, scientists require the ability to easily search through data, ask it questions, get visualization and analysis, all with full scientific context. Equally important is the need to do this right where they already work, without requiring custom code, time consuming workarounds, multiple logins, or an army of data scientists. It also helps if the data is stored in a way that allows it to be used to train the ML models scientists are increasingly using.
Only a solution that bridges the gap between consolidation and utilization can solve the data problem for today’s scientists. This type of solution is built to make life easier for scientists, drive productivity, accelerate discovery workflows, and support scientific needs as they evolve.
Making the business case for the science-aware approach
For most scientific organizations, a world of manual instrument exports, data warehouses, and siloed lab informatics tools is ‘simply the way it’s done.’
But this laborious, fragmented approach to scientific data management has a real impact on the pace of scientific research projects and discovery. And the question scientific organizations should be asking themselves is ‘how much time are my scientists losing by not having an easy way to search, analyze and visualize the scientific data they rely on?’
Let’s consider a hypothetical scenario that should be familiar to many scientists. A scientist in a core facility (say an analytical chemistry lab) spends 16 hours each week collecting and processing data from different systems and instruments, 8 hours analyzing data, and another 4 hours compiling meaningful reports. That’s 28 hours of an average 40-hour work week, or 70% of the scientist’s time spent on data collection and analysis alone.
While you don’t need to take a science-aware approach to reduce the time required to collect and process data to 0, that’s only half of the problem in this example. It is analyzing the data and compiling reports that also eats into the scientists’ time. But with a science-aware approach to data consolidation, reports are automatically generated, and the time spent analyzing data is reduced significantly as well. The collective time savings can reduce what was 28 hours to just 6 hours, with those 6 hours dedicated to the highest value analytical tasks that make the greatest impact on scientific progress.
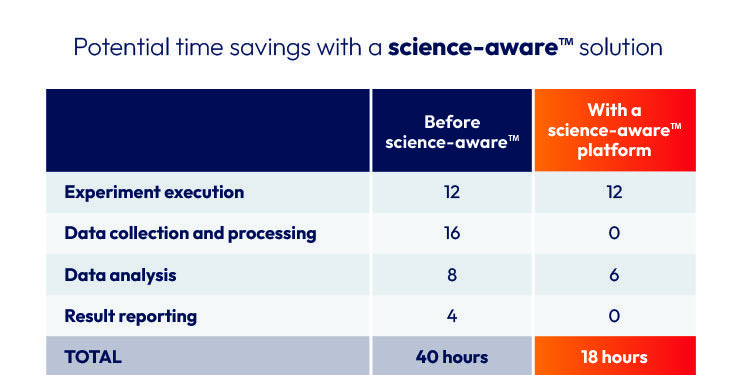
These time savings are powerful on a small scale, but over the course of a year and with multiple scientists, they are even more significant. Imagine the impact on productivity and scientific progress if more than half of a scientist’s average work week was reallocated from gathering and analyzing data to focusing on scientific discovery?
Because a science-aware solution is made for the unique data and system demands of scientists and scientific organizations, it is much easier for scientists themselves to use. Scientists are confident in the benefits, and an interface that works the way they do mitigates lengthy training and delayed adoption.
If you’re interested in learning more, we’ve taken a look at some of the common scenarios where there’s a clear business case for taking a science-aware approach to consolidating your lab data:
- Optimizing Data Collection and Analysis in Scientific Research
- How To Make Your Scientific Data Less Fragmented
- Build vs Buy: What’s Better for Lab Instrument Data Integration?
Jarvis: The only data platform built for scientists
Sapio Jarvis is the first truly science-aware™ data platform, built from the ground up for scientists. With Jarvis’ powerful data standardization, intuitive interface, integrated analytics, and AI-enabled chat capabilities, scientists can virtually eliminate the time spent looking for data across instruments and easily search, visualize, and analyze their data in a way that tangibly advances the speed and quality of their science.
If you’d like a demo of Jarvis or are ready to take the next step, speak to an expert.
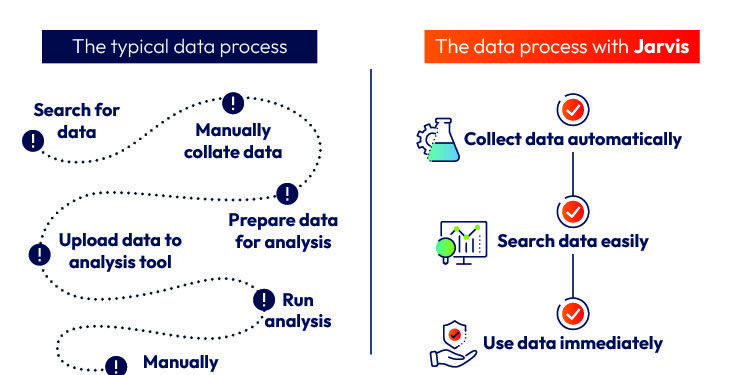